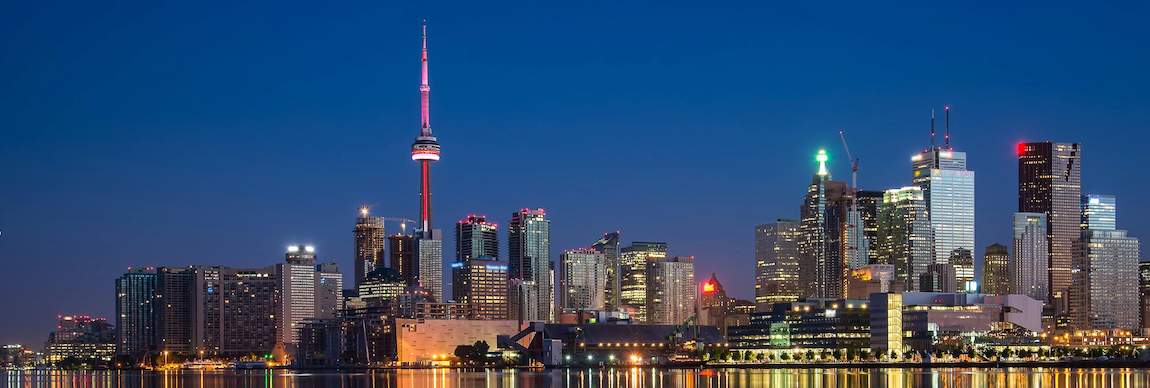
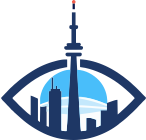
TORONTO VISION SEMINAR
The Toronto Vision Seminar is a monthly seminar series in computer vision that aims to (1) gather academic and industry researchers in vision from the Greater Toronto Area and (2) strengthen ties between researchers within the vision community in Canada and internationally. Seminar talks are delivered primarily in person by domestic and international researchers from academia and industry. Seminar topics span the areas of computer vision, applied mathematics, optimization, and machine learning and may include, for example, perception, generative modeling, neural representations, 3D reconstruction, computational photography, plenoptic imaging, and light transport analysis. Attendees include university students and researchers, faculty, and industry researchers.
Organizers
Sponsors
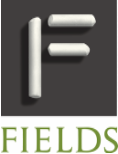
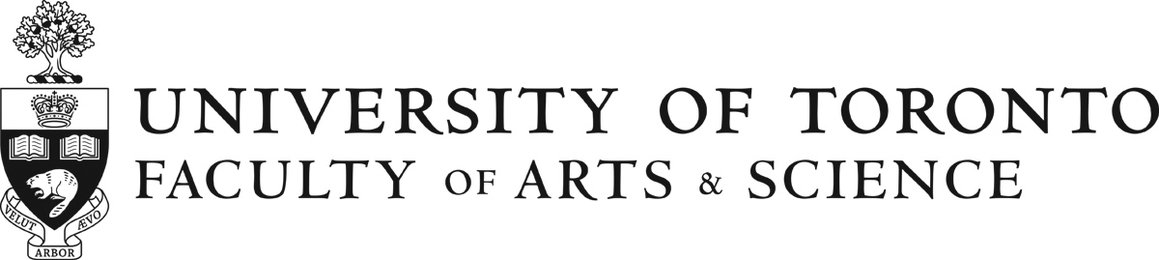
2024–2025 Schedule
Srinivas Narasimhan (Carnegie Mellon University)
April 11th
“Computer Vision with Light, Sound, and Heat”
In recent years, computer vision has made remarkable strides, yet it remains largely confined to what our eyes can see. In this talk, we step into a world beyond the visible spectrum, where light, sound, and heat converge to unlock new possibilities in computer vision. By harnessing ultrasound to control light, we can image deeper and with higher resolution, overcoming tissue scattering and enable ultra-fast optical scanning without moving parts. By examining the interplay between light and heat transport, we can reconstruct object shapes regardless of their visible reflectances and scene lighting, resolving long-standing ambiguities in physics-based vision. By seeing extremely tiny vibrations, we turn our visual cameras into audio and mechanical sensors. Our research has practical applications across various scientific domains, from detecting cancerous tumors to measuring plant photosynthesis to material defect detection. These research projects are a result of collaborations with my fantastic faculty colleagues, postdocs and students.
Aaron Hertzmann (Principal Scientist, Adobe Research)
April 2nd
“Toward a Theory of Perspective Perception in Pictures”
I propose a new approach to understanding how human vision interprets 3D shape in realistic pictures, specifically focusing on perspective. I argue that most of human shape perception happens in single eye fixations, mostly in foveal vision, and that humans preserve surprisingly little 3D awareness over time. In pictures, this meanst that each individual eye fixation can have, to some extent, a separate perspective from the others. This theory integrates ideas from human vision science, art history, and computational photography, and suggests new ways to think about how pictures work, and how we can make pictures. In addition, I will briefly outline a more general theory around how picture perception works, accounting for phenomena like tone-mapping (which is present, in some form, in all realistic pictures) and various stylization techniques.
Andrea Tagliasacchi (Simon Fraser University)
February 19th
“3D Computer Vision and its evolution from depth sensors to novel view synthesis”
Over the last decade, computer vision has undergone a remarkable transformation with many difficult problems now considered solved. However, it is important to recognize that much of this progress is often confined to 2D images and videos, which only provide superficial understanding of the underlying 3D structure. Achieving a comprehensive understanding of 3D scenes remains largely an unsolved challenge. Solving this problem is crucial, as computer vision shifts from passive tasks, like search and surveillance, to more active applications that require 3D modeling, such as those that drive the decision-making process of embodied autonomous systems that interact with the 3D environment. In this talk, I will present my research journey through these areas, beginning with real-time processing techniques for Kinect-style sensors in computer graphics leading to the development of neural 3D
representations, and their unsupervised training through “novel-view synthesis” objectives.
Roberto Abraham (University of Toronto)
November 27th
“Crazy Telescopes, Ghostly Galaxies, and the Invisible Universe”
Bigger telescopes are usually better telescopes… but not always. Sometimes crazier telescopes are better telescopes. In this talk I will describe the nearly unexplored universe of ghostly, nearly undetectable phenomena in the heavens, and describe how mosaic telescope arrays are being used to open up this new area of astrophysics. I will focus on why finding these “low surface brightness” objects is important, and why it has also been so devilishly difficult to find them using “normal” telescopes. We have probably been missing out seeing a vast range of exotic objects, like low-surface brightness dwarf galaxies, supernova light echoes, galactic halos, and planetary dust rings. These objects are nearly undetectable with conventional telescopes, but their properties may hold the keys to understanding a host of fundamental phenomena, including the nature of dark matter and the mechanisms by which galaxies form and evolve. These things are hard to study, but bizarre new telescopes, made possible by technological advances driven by mobile phone camera sensors and processors, and ubiquitous access to fast networks, are changing the landscape. The Dragonfly Telephoto Array (a.k.a. Dragonfly) is an example of this new class of telescope. Dragonfly is comprised of 168 off-the-shelf high-end telephoto lenses utilizing novel nanostructure-based optical coatings. I will showcase some early results from Dragonfly, and describe how this array is evolving to tackle the ultimate challenge in this subject: directly imaging the “Cosmic Web”. This is the largest collapsed structure in the Universe, and the repository of most of its matter. We know this web exists, but nobody knows what it really looks like, or how it funnels the gas created by the Big Bang into pockets of dark matter to drive the formation of galaxies. We are now building a massive expansion of the Dragonfly telescope that will let us take pictures of the Cosmic Web, and we hope to find these things out.
Dima Damen (University of Bristol)
November 22nd
“Opportunities in Egocentric Vision”
Forecasting the rise or wearable devices equipped with audio-visual feeds, this talk will present opportunities for research in egocentric video understanding. The The talk argues for new ways to foresee egocentric videos as partial observations of a dynamic 3D world, with objects out of sight but not out of mind. I’ll review new data collection and annotation that merges video understanding with 3D modelling, showcasing current failures of VLMs in understanding the perspective outside the camera’s field of view — a task trivial for humans.
Kristina Monakhova (Cornell University)
October 16th
“Trustworthy and adaptive extreme low light imaging”
Imaging in low light settings is challenging due to low photon counts. In photography, imaging under low light, high gain settings often results in highly structured, non-Gaussian noise that’s hard to characterize or denoise. In scanning microscopy, the push to image faster, deeper, with less damage, and for longer durations, can result in noisy measurements and less signal acquired. In this talk, we’ll address three problems in denoising that are important for real applications: 1) What can you do when your noise is sensor-specific and non-Gaussian? 2) How can you trust the output of a denoiser enough for critical scientific and medical applications? and 3) If you can sample a noisy scene multiple times, which parts should you resample? For the first problem, I’ll introduce a sensor-specific, data-driven, physics-inspired noise model for simulating camera noise at the lowest light and highest gain settings. I’ll then use this noise model as a building block for demonstrating photorealistic videography by the light of only the stars (submillilux levels of illumination). Next, I’ll introduce an uncertainty quantification technique based on conformal prediction to simultaneously denoise and predict the pixel-wise uncertainty in microscopy images. Then, I’ll use uncertainty-in-the-loop to drive adaptive acquisition for scanning microscopy, reducing the total scan time and light dose to the sample, while minimizing uncertainty.
Michal Irani (Weizmann Institute)
September 13th
“Deep Internal learning” – Deep Learning without prior examples
In this talk I will show how Deep-Learning can be performed without any prior examples, by training on a single image/video – the test input alone. The strong recurrence of information inside a single natural image/video provides powerful internal examples which suffice for self-supervision of Deep-Networks, thus giving rise to true “Zero-Shot Learning”. I will show the power of this approach to a variety of problems, including: super-resolution (in space and in time), image-segmentation, transparent layer separation, image-dehazing, diverse image/video generation, and more.
2023–2024 Schedule
Todd Zickler (Harvard University)
May 8th
Images as Fields of Junctions
Which pixels belong together, and where are the boundaries between them? My talk revisits these enduring questions about grouping, this time informed by the apparent strengths and weaknesses of modern foundation models. I focus on exactness and generality, aiming to localize boundaries with high spatial precision by exploiting low-level geometric consistencies between boundary curves, corners and junctions. Our approach represents the appearance of each small receptive field by a low-parameter, piece-wise smooth vector-graphics model (a “generalized junction”), and it iteratively decomposes an image into a dense spatial field of such models. This decomposition reveals precise edges, curves, corners, junctions, and boundary-aware smoothing—all at the same time. I present experiments showing this provides unprecedented resilience to image degradations, producing stable output at high noise levels where other methods fail. I also discuss recent work that accelerates the decomposition using a specialized form of spatial self-attention. At the open and close of the talk, I speculate about how these capabilities may help us close gaps between mid-level vision in animals and machines.
Katerina Fragkiadaki (Carnegie Mellon University)
April 10th
Systems 1 and 2 for Robot Learning
Humans can successfully handle both easy (mundane) and hard (new and rare) tasks simply by thinking harder and being more focused. In contrast, today’s robots spend a fixed amount of compute in both familiar and rare tasks, that lie inside and far from the training distribution, respectively, and do not have a way to recover once their fixed-compute inferences fail. How can we develop robots that think harder and do better on demand? In this talk, we will marry today’s generative models and traditional evolutionary search and 3D scene representations to enable better generalization of robot policies, and the ability to test-time think through difficult scenarios, akin to a robot system 2 reasoning. We will discuss learning behaviours through language instructions and corrections from both humans and vision-language foundational models that shape the robots’ reward functions on-the-fly, and help us automate robot training data collection in the simulator and in the real world. The models we will present achieve state-of-the-art performance in RLbench, Calvin, nuPlan, Teach, and Scannet++, which are established benchmarks for manipulation, driving, embodied dialogue understanding and 3D scene understanding.
Ellen Zhong (Princeton University)
November 22nd
Machine learning for determining protein structure and dynamics from cryo-EM images
Major technological advances in cryo-electron microscopy (cryo-EM) have produced new opportunities to study the structure and dynamics of proteins and other biomolecular complexes. However, this structural heterogeneity complicates the algorithmic task of 3D reconstruction from the collected dataset of 2D cryo-EM images. In this seminar, I will overview cryoDRGN and related methods that leverage the representation power of deep neural networks for cryo-EM reconstruction. Underpinning the cryoDRGN method is a deep generative model parameterized by an implicit neural representation of 3D volumes and a learning algorithm to optimize this representation from unlabeled 2D cryo-EM images. Extended to real datasets and released as an open-source tool, these methods have been used to discover new protein structures and visualize continuous trajectories of protein motion. I will discuss various extensions of the method for scalable and robust reconstruction, analyzing the learned generative model, and visualizing dynamic protein structures in situ.