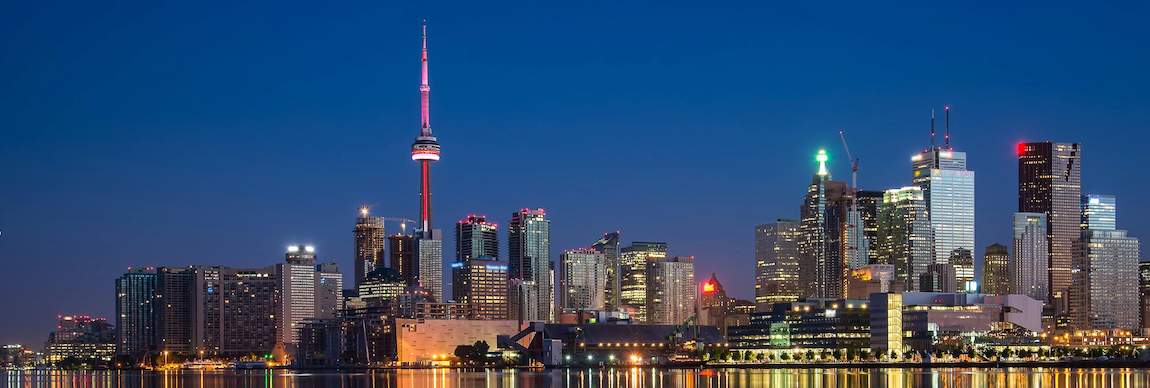
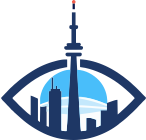
TORONTO VISION SEMINAR
The Toronto Vision Seminar is a monthly seminar series in computer vision that aims to (1) gather academic and industry researchers in vision from the Greater Toronto Area and (2) strengthen ties between researchers within the vision community in Canada and internationally. Seminar talks are delivered primarily in person by domestic and international researchers from academia and industry. Seminar topics span the areas of computer vision, applied mathematics, optimization, and machine learning and may include, for example, perception, generative modeling, neural representations, 3D reconstruction, computational photography, plenoptic imaging, and light transport analysis. Attendees include university students and researchers, faculty, and industry researchers.
Organizers
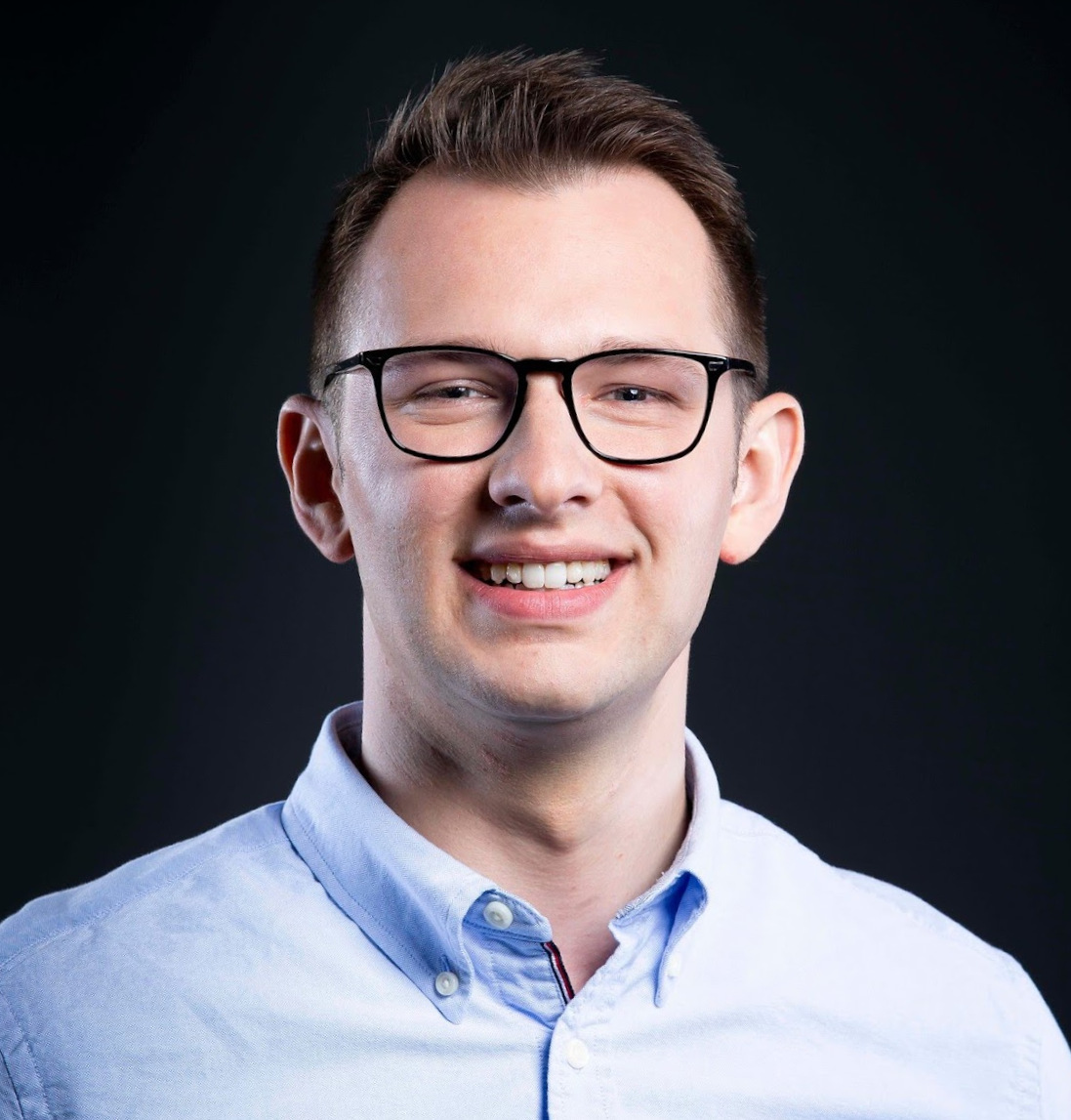
University of Toronto

York University
Sponsors
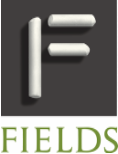
2023–2024 Schedule
Todd Zickler (Harvard University)
Images as Fields of Junctions
Which pixels belong together, and where are the boundaries between them? My talk revisits these enduring questions about grouping, this time informed by the apparent strengths and weaknesses of modern foundation models. I focus on exactness and generality, aiming to localize boundaries with high spatial precision by exploiting low-level geometric consistencies between boundary curves, corners and junctions. Our approach represents the appearance of each small receptive field by a low-parameter, piece-wise smooth vector-graphics model (a “generalized junction”), and it iteratively decomposes an image into a dense spatial field of such models. This decomposition reveals precise edges, curves, corners, junctions, and boundary-aware smoothing—all at the same time. I present experiments showing this provides unprecedented resilience to image degradations, producing stable output at high noise levels where other methods fail. I also discuss recent work that accelerates the decomposition using a specialized form of spatial self-attention. At the open and close of the talk, I speculate about how these capabilities may help us close gaps between mid-level vision in animals and machines.
Katerina Fragkiadaki (Carnegie Mellon University)
Systems 1 and 2 for Robot Learning
Humans can successfully handle both easy (mundane) and hard (new and rare) tasks simply by thinking harder and being more focused. In contrast, today’s robots spend a fixed amount of compute in both familiar and rare tasks, that lie inside and far from the training distribution, respectively, and do not have a way to recover once their fixed-compute inferences fail. How can we develop robots that think harder and do better on demand? In this talk, we will marry today’s generative models and traditional evolutionary search and 3D scene representations to enable better generalization of robot policies, and the ability to test-time think through difficult scenarios, akin to a robot system 2 reasoning. We will discuss learning behaviours through language instructions and corrections from both humans and vision-language foundational models that shape the robots’ reward functions on-the-fly, and help us automate robot training data collection in the simulator and in the real world. The models we will present achieve state-of-the-art performance in RLbench, Calvin, nuPlan, Teach, and Scannet++, which are established benchmarks for manipulation, driving, embodied dialogue understanding and 3D scene understanding.
Ellen Zhong (Princeton University)
Machine learning for determining protein structure and dynamics from cryo-EM images
Major technological advances in cryo-electron microscopy (cryo-EM) have produced new opportunities to study the structure and dynamics of proteins and other biomolecular complexes. However, this structural heterogeneity complicates the algorithmic task of 3D reconstruction from the collected dataset of 2D cryo-EM images. In this seminar, I will overview cryoDRGN and related methods that leverage the representation power of deep neural networks for cryo-EM reconstruction. Underpinning the cryoDRGN method is a deep generative model parameterized by an implicit neural representation of 3D volumes and a learning algorithm to optimize this representation from unlabeled 2D cryo-EM images. Extended to real datasets and released as an open-source tool, these methods have been used to discover new protein structures and visualize continuous trajectories of protein motion. I will discuss various extensions of the method for scalable and robust reconstruction, analyzing the learned generative model, and visualizing dynamic protein structures in situ.